2023.11.27
Press Release
Unveiling New Risks Lurking in the High Performance of Artificial Intelligence: Anticipating Enhanced Reliability in Medical Image Diagnosis
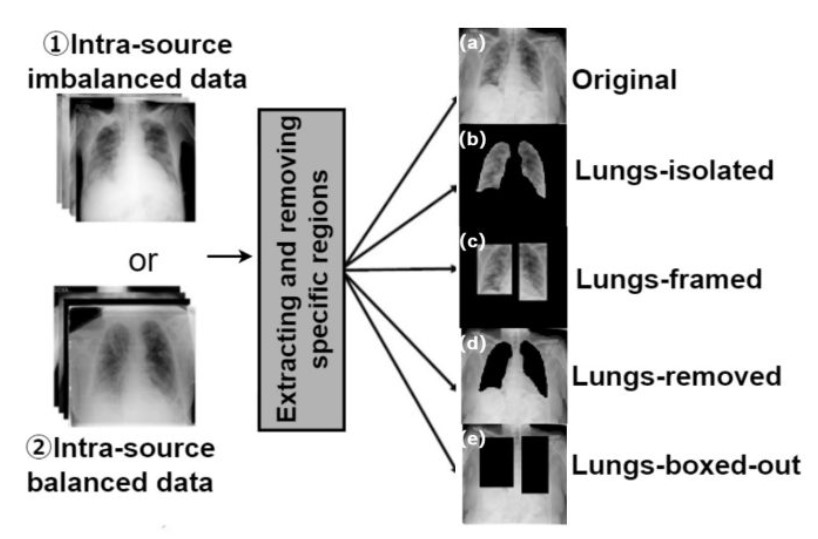
With the advancement of artificial intelligence (AI) technology, particularly in the realm of deep learning, there is growing interest in its application for medical tasks. Deep learning requires a substantial amount of training data, presenting challenges in data acquisition. Specifically, the characteristics of training data significantly influence the performance of AI. For example, an issue named class imbalance, which means an imbalance in data number between disease and normal groups, can lead to decreased performance. For avoiding this issue, in situations where urgent cases like the diagnosis of COVID-19 make it difficult to secure sufficient data, efforts have been made to balance the number of disease and normal cases by collecting data from several hospitals. While this approach may seem to alleviate the class imbalance issue, it has been observed that a new problem, that developed AI can appear to be high-performing but may actually be poor-performing (Image 1).
The research group from the Department of Radiological Imaging and Informatics, Tohoku University Graduate School of Medicine, which includes Researcher Zhang Zhang, Associate Professor Xiaoyong Zhang (Current Affiliation: The Department of General Engineering, National Institute of Technology, Sendai college), and Professor Noriyasu Homma, have newly identified the cause of this performance decline, termed “intra-source imbalance”. Intra-source imbalance occurs when the AI inadvertently learns differences in data sources, such as the hospitals or devices used for imaging, which are unrelated to the medical differences between disease and normal groups. Using the example of COVID-19 diagnosis with chest X-ray images, our research looked into the impact of intra-source imbalance on the performance of deep learning. To confirm whether the model is learning medical differences, they performed image processing by hiding or cropping important parts of the lungs for diagnosis on an intra-source balanced dataset and an intra-source imbalanced dataset separately, to create separate datasets for each processing step (Image 2). The results of our experiments demonstrated a decrease in reliability when models were trained on intra-source imbalanced data. Additionally, when comparing the regions of interest highlighted by the model using visualization methods, the intra-source balanced data-trained model focused on more medically relevant areas (Image 3). This finding underscores the significance of addressing intra-source imbalance in training data of AI models, which will lead to the development of trustworthy AI in the field of healthcare in the future.
Details of their research were published in the journal Scientific Reports on November 3, 2023
Publication Details:
Title: How intra-source imbalanced datasets impact the performance of deep learning for COVID-19 diagnosis using chest X-ray images
Authors: Zhang Zhang, Xiaoyong Zhang, Kei Ichiji, Ivo Bukovský and Noriyasu Homma
Journal: Scientific Reports
DOI: doi.org/10.1038/s41598-023-45368-w
https://www.nature.com/articles/s41598-023-45368-w
Contact:
Name: Zhang ZHANG, Noriyasu HOMMA
Affiliation: Department of Radiological Imaging and Informatics, Tohoku University Graduate School of Medicine
Email: zhangzhang*tohoku.ac.jp Please replace “*” with “@”.
homma*med.tohoku.ac.jp Please replace “*” with “@”.
Website: https://www.rii.med.tohoku.ac.jp/
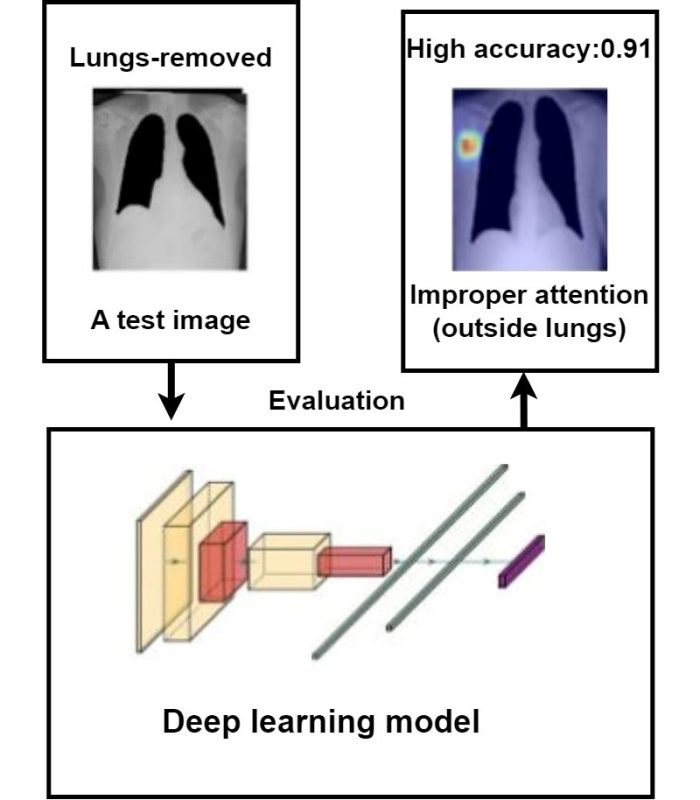
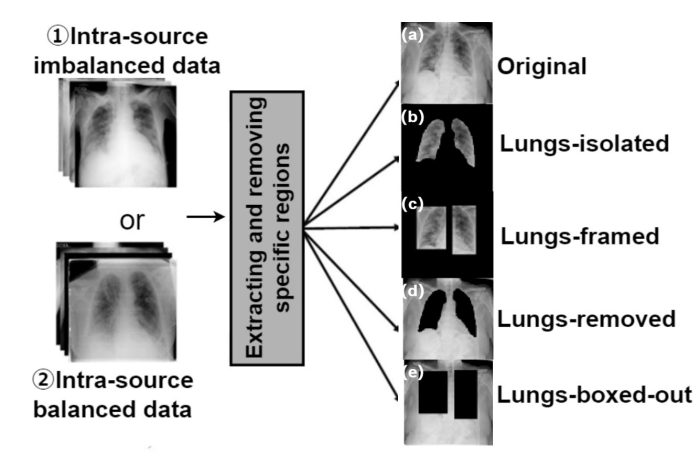
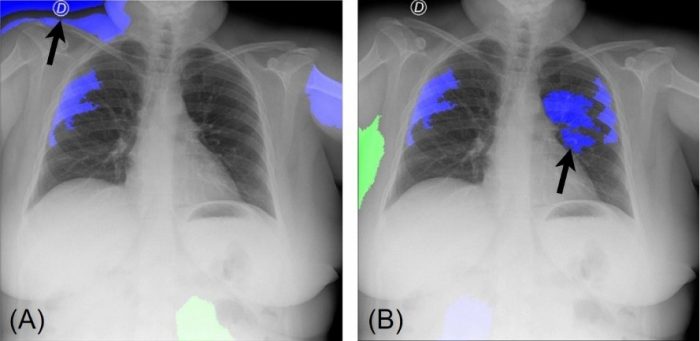